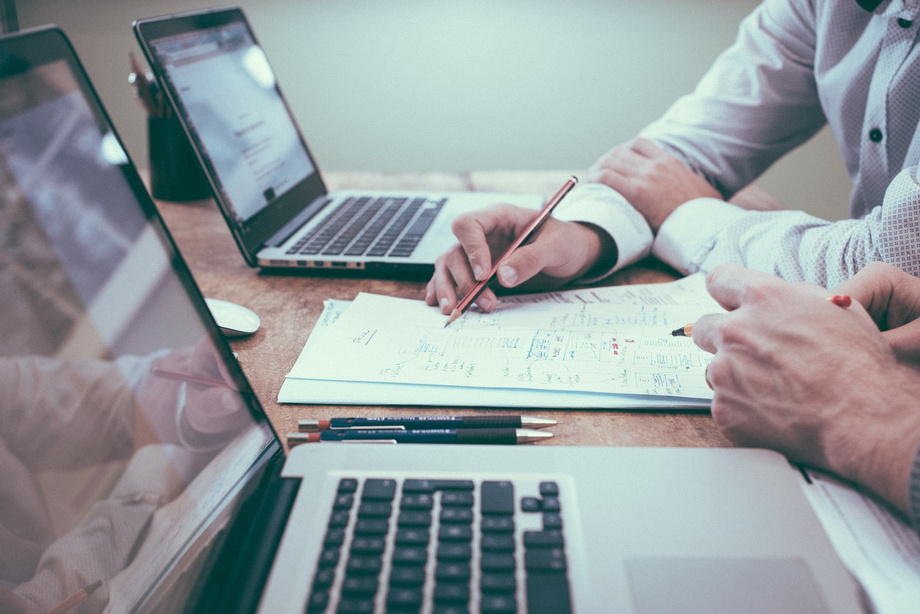
Free Photos, Pixabay, CC0
Background
Current day algorithms governing how medical devices deliver stimulation to patients have not changed in well over 50 years and cannot account for neurological variabilities across individuals that come about from differences in genetics, lifestyle factors, and sustained injuries. As a result, clinicians are experiencing highly inconsistent patient outcomes, with 30%-90% of individuals not responding to treatment at all, depending on the disease. A large quantity of research in the fields of computational and systems neuroscience has demonstrated that the clinical state-of-the-art is insufficient to remedy many pathologies and must be re-invented for better results.
Technology
Escape-the-Maze (EtM), is a brand-new type of reinforcement learning algorithm. EtM is the first of its kind and is installed on existing medical devices in a closed-loop recording-stimulating setup with the brain circuit of interest, where it leverages the patient’s unique neural activity to guide the electrical activity out of the unwanted state e.g coma. Due to EtM’s versatility, there are a wide array of applicable markets associated with neuropsychiatric and movement disorders such as obsessive compulsive disorder (OCD), as well as disorders of consciousness.
Advantages
Personalized algorithms to treat various disorders - Automatically modulates stimulus applied by medical devices - Hardware agnostic (both invasive and non-invasive treatments)
Application
Treatment of neuropsychiatric, movement, and consciousness disorders.
Inventors
Ian Jordan, , Department of Applied Mathematics and Statistics
Il Park, Assistant Professor, Neurobiology and Behavior
Josue Nassar, , Electrical and Computer Engineering
Licensing Potential
Development partner - Commercial partner - Licensing
Licensing Status
Available
Licensing Contact
Valery Matthys, Licensing Associate, Intellectual Property Partners, valery.matthys@stonybrook.edu,
Patent Status
Patent Application Published: WO2023/196280
In-silico data
Tech Id
050-9281